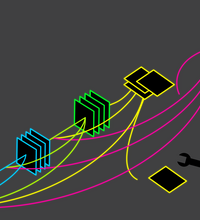
A New Perspective on Adversarial Perturbations
Aleksander Madry (MIT)
The widespread susceptibility of the current ML models to adversarial perturbations is an intensely studied but still mystifying phenomenon. A popular view is that these perturbations are aberrations that arise due to statistical fluctuations in the training data and/or high-dimensional nature of our inputs.
But is this really the case?
In this talk, I will present a new perspective on the phenomenon of adversarial perturbations. This perspective ties this phenomenon to the existence of "non-robust" features: features derived from patterns in the data distribution that are highly predictive, yet brittle and incomprehensible to humans. Such patterns turn out to be prevalent in our real-world datasets and also shed light on previously observed phenomena in adversarial robustness, including transferability of adversarial examples and properties of robust models. Finally, this perspective suggests that we may need to recalibrate our expectations in terms of how models should make their decisions, and how we should interpret them.