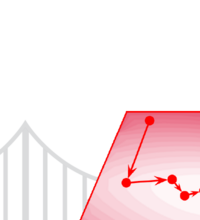
Subdeterminant Maximization via Nonconvex Relaxations and Anti-concentration
The problem of subdeterminant maximization subject to combinatorial constraints arises in various algorithmic and machine learning settings and, recently, convex-programming based estimation algorithms for it have been proposed. The question of whether these estimation algorithms can be converted into the more useful approximation algorithms -- that also output a set -- remained open.
We present novel nonconvex formulations for the constrained subdeterminant maximization problem and prove a new anti-concentration inequality for dependent random variables that together allow us to obtain approximation algorithms for this problem in a variety of settings.
Based on a joint work with Javad Ebrahimi and Damian Straszak available https://arxiv.org/abs/1707.02757