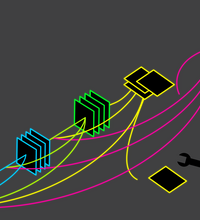
Reinforcement Learning in Recommender Systems: Some Challenges
Craig Boutilier (Google and University of Toronto)
I'll present a brief overview of some recent work on reinforcement learning motivated by practical issues that arise in the application of RL to online, user-facing applications like recommender systems. These include (a) stochastic action sets; (b) long-term cumulative effects; and (c) combinatorial action spaces. With respect to (c) I will discuss SlateQ, a novel decomposition technique that allows value-based RL (e.g., Q-learning) in slate-based recommender to scale to commercial production systems, and briefly describe both small-scale simulation and a large-scale experiment with YouTube. With respect to (b), I will briefly discuss a Advantage Amplification, a temporal aggregation technique that allows for more effective RL in partially observable domains with low SNR, as often arise in recommender systems.