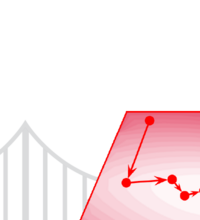
The Power and Limitations of Kernel Learning
In the last few years we have seen remarkable progress in machine learning in large part due to neural networks trained on large data. While shallow methods, in particular methods based on positive definite kernels, show excellent results on smaller data sets, their performance suffers at large scale. It turns out that some of this lack of performance can be attributed to the computational limitations of kernel learning. The span of smooth kernel functions (such as Gaussians) is dense in the space of all functions and theoretically any function can be approximated arbitrarily well. However, the space of functions reachable within a fixed computational budget is quite limited. This can be formalized in terms of the fat shattering dimension and other measures of complexity. This computational barrier turns out to be related to the smoothness of the kernel and to the types of computations available for large data. One way to address this issue is by constructing new, less smooth kernels, designed for efficient computation. The resulting algorithms show significant improvements over the state of the art in large scale kernel methods, typically at a fraction of the computational budget reported in the literature. Finally, we hope that deeper understanding of kernel-based learning can also shed light on the success of neural networks.
Based on joint work with Siyuan Ma.
Attachment | Size |
---|---|
![]() | 1.17 MB |