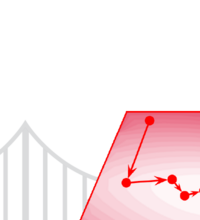
Online Optimization, Smoothing, and Competitive Ratio
In Online Optimization, the data in an optimization problem is revealed over time, and at each step a decision variable needs to be set without knowing the future data. We consider an online optimization setup that includes problems such as online resource allocation with a fixed inventory and the `Adwords' problem popular in online advertising. We consider two broad primal-dual algorithms, with a focus on the competitive ratio, i.e., the ratio of the objective achieved by the algorithm to that of the optimal offline sequence of decisions. We give a bound on this ratio and show how certain smoothing of the objective function can improve the bound; and for separable functions, how to seek the optimal smoothing by solving a convex design problem. This allows us to design effective smoothing customized for a given cost function and problem structure.