Talks
Fall 2017
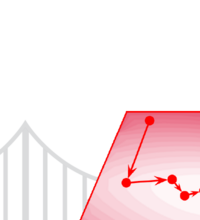
On Approximation Guarantees for Greedy Low Rank Optimization
Thursday, November 30th, 2017, 11:00 am–11:30 am
Speaker:
We provide new approximation guarantees for greedy low rank matrix estimation under standard assumptions of restricted strong convexity and smoothness. Our novel analysis also uncovers previously unknown connections between the low rank estimation and combinatorial optimization, so much so that our bounds are reminiscent of corresponding approximation bounds in submodular maximization. Additionally, we provide also provide statistical recovery guarantees. Finally, we present empirical comparison of greedy estimation with established baselines on two real-world problems.