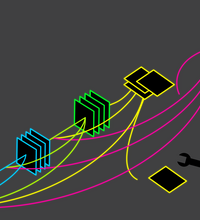
Improving medical predictions by caring about uncertainty: Combining Deep Neural Networks with Bayesian methodology
Katherine Heller
Room 116
The first part of this talk focuses on the concrete example of combining Gaussian Process (GPs) with Recurrent Neural Networks (RNNs) for the purpose of predicting sepsis in hospital patients. We show that in this example, capturing uncertainty by sampling from the GP results in better predictions than alternatives which do not account for this uncertainty.
We describe this model's deployment into the Emergency Department of a major hospital as motivation for how the accuracy of these predictions affects the lives of patients right now.
We then begin to thoroughly examine uncertainty within a medical context. When does uncertainty make a difference? What kind of uncertainty matters? How is this measured? What effect might this have on underrepresented patient groups? The integration of Bayesian techniques with deep neural networks for the purposes of patient mortality prediction provides a setting in which we try to answer these questions.