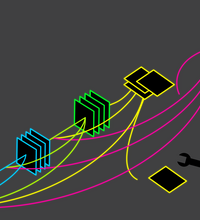
Reinforcement Learning in Feature Space: Complexity and Regret
Mengdi Wang (Princeton University)
Recent years have witnessed increasing empirical successes in reinforcement learning (RL). However, many theoretical questions about RL are not well understood even in the most basic setting. For example, how many observations are needed and sufficient for learning a good policy? How to learn to control in unstructured Markov decision process with provable regret? In this talk, we study the statistical efficiency of reinforcement learning in feature space and show how to learn the optimal policy algorithmically and efficiently. We will introduce feature-based reinforcement learning algorithms with minimax-optimal sample complexity and near-optimal regret. We will also discuss a state embedding learning method that is able to automatically learn state features from state trajectories.
Attachment | Size |
---|---|
![]() | 9.44 MB |