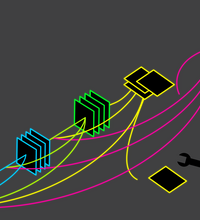
Reducing AI Bias using Truncated Statistics
Constantinos Daskalakis
Room 116
An emergent threat to the practical use of machine learning is the presence of bias in the data used to train models. Biased training data can result in models which make incorrect, or disproportionately correct decisions, or that reinforce the injustices reflected in their training data. For example, recent works have shown that semantics derived automatically from text corpora contain human biases, and found that the accuracy of face and gender recognition systems are systematically lower for people of color and women.
While the root causes of AI bias are difficult to pin down, a common cause of bias is the violation of the pervasive assumption in machine learning and statistics that the training data are unbiased samples of an underlying “test distribution,” which represents the conditions that the trained model will encounter in the future. We present a practical framework, based on SGD and truncated statistics, for regression and classification targeting such settings, which identifies both the mechanism inducing the discrepancy between the training and test distributions, and a predictor that targets performance in the test distribution. Our framework provides computationally and statistically efficient algorithms for truncated density estimation and truncated linear, logistic and probit regression. We provide experiments to illustrate the efficacy of our framework in removing bias from gender classifiers.
(Based on joint works with Themis Gouleakis, Andrew Ilyas, Vasilis Kontonis, Sujit Rao, Christos Tzamos, Manolis Zampetakis)